Key Takeaways:
- ➢ AI’s growing computational demand requires infrastructure investors to prioritize energy-efficient data centers and digital infrastructure.
- ➢ AI is effective in narrow tasks, but long-term infrastructure investments demand human judgment and a deep understanding of complex, domain-specific nuances for strategic planning.
- ➢ Preserving institutional knowledge in an aging infrastructure workforce will rely on integrating AI with human expertise to effectively navigate the complexities of long-term infrastructure investments.
The rapid advancements in AI technology are fundamentally transforming industries, extending far beyond the digital realm into the very core of the infrastructure that powers our physical and virtual worlds. AI models, such as ImageNet-based computer vision systems and large language models (LLMs), have experienced unprecedented growth in performance and complexity, driven by substantial investments in data processing, model training, and computational infrastructure. This convergence of digital and physical infrastructure is reshaping how infrastructure projects are planned, executed, and maintained, while also presenting new challenges and opportunities for long-term investors.
This article examines two interrelated points: first, the critical role of infrastructure in enabling AI progress, and second, the implications of AI’s development for strategic decision-making in the infrastructure sector. While AI excels in optimizing short-term operational tasks, its application to long-term strategic planning—crucial for infrastructure investments—is limited by challenges such as uncertainty, reasoning, complexity, and multi-stakeholder dynamics. This article explores how AI can help address these nuanced, domain-specific challenges and support human experts in solving them.
AI can contribute at both micro and macro levels—by providing detailed project-specific insights and by informing a broader understanding of the infrastructure domain, stakeholders, and evolving landscape. Underlying all this is the critical importance of a robust data ecosystem for infrastructure construction and domain knowledge encoding for AI systems.
AI’s Infrastructure Dependency – Data Centers and Computational Capacity
The rapid advancements in AI, particularly in large-scale tasks such as image recognition and language modeling, have been fueled by significant investments in computational infrastructure. For example, training OpenAI’s GPT-4 cost an estimated $78 million, while Google’s Gemini Ultra reached $191 million in compute costs. These figures underscore the growing demand for robust, energy-efficient data centers capable of supporting massive AI workloads.
This trend has significant implications for infrastructure investors, who must now consider high-performance data centers as elements of their infrastructure portfolios. As AI evolves, energy and transportation infrastructure must increasingly integrate with digital demands, driving a new wave of investment in both physical and digital infrastructure.
Another critical consideration is the democratization of access to AI infrastructure. As training costs for advanced AI models skyrocket, smaller institutions, particularly academic research centers, find themselves priced out of cutting-edge AI research. This has led to calls for policy interventions, such as President Biden’s Executive Order on AI, which aims to establish a National AI Research Resource to provide equitable access to compute and data for non-industry actors. Policymakers and infrastructure investors alike will play a vital role in ensuring that AI infrastructure remains accessible and fosters innovation across sectors.
While compute power often dominates discussions of AI infrastructure, data infrastructure is equally critical to the continued advancement of AI. The foundation of AI training lies in massive datasets, and the scale of data required has expanded at a remarkable rate. For example, ImageNet revolutionized computer vision by leveraging millions of labeled images, while LLMs require a vast corpora of text data. For the infrastructure domain, curated data about “bread and butter” information about projects and related risks is a key constraint in building large-scale AI systems for infrastructure construction.
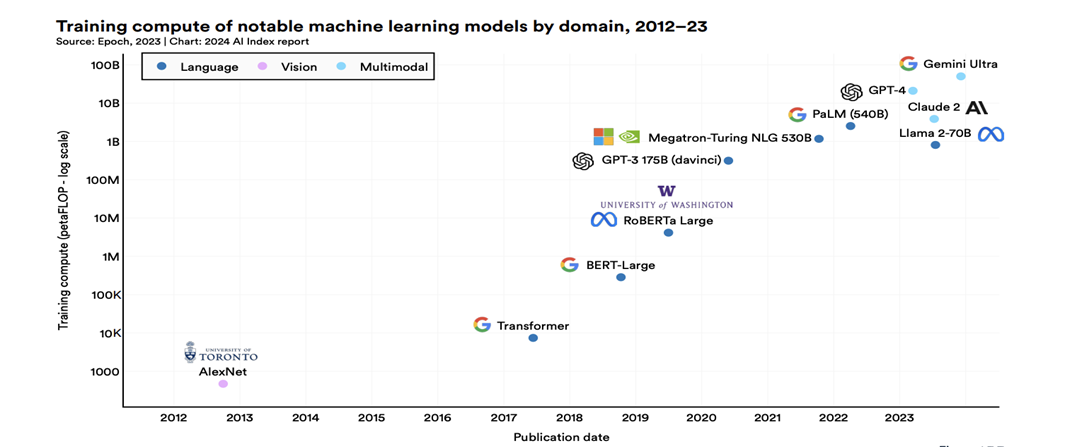
AI Applications and Long-term Infrastructure Investment Decisions
Despite its success in optimizing short-term operations and mechanical tasks, AI remains limited in its ability to support long-term infrastructure planning. Projects that span 30-50 years are influenced by a range of complex factors including demographic changes, economic cycles, and climate risks. While AI excels at narrow tasks, it struggles with the uncertainty, reasoning, and complexity inherent in long-term strategic decision-making.
For instance, AI lacks the ability to factor in dynamic socio-political changes, emerging market trends, and unpredictable environmental shifts, all of which are critical to long-term infrastructure planning. While creative applications of AI in long-range planning, urban design, and metro system designs are promising, they are still in their nascent stages.
AI’s limitations in this domain stem from its reliance on historical data and difficulties in reasoning about uncertain or evolving environments. Infrastructure investments require careful long-term decision-making that cannot be entirely delegated to AI systems. However, AI can assist by producing new insights, performing mechanical tasks, and helping to understand stakeholder dynamics and evolving markets—relieving investors and stakeholders of the burden of data collection and research.
Building the Future of Infratech
The intersection of AI and infrastructure presents both challenges and opportunities. While AI has made significant strides in task-specific performance, its application to long-term strategic planning for infrastructure remains limited by uncertainty, reasoning capabilities, market knowledge, and the complexity of possible future scenarios.
For infrastructure investors, the key takeaway is that AI can be a powerful tool for short-term operational improvements and risk management, particularly through predictive analytics and simulations – traditional ML systems already in use over the last decade. However, the long-term success of infrastructure projects will depend on a hybrid approach that combines AI’s data-driven insights with human judgment and experience. The future of Infratech will be shaped by those who can effectively integrate the digital and physical worlds, using AI to navigate the complexities of long-term planning while addressing the immediate demands of infrastructure performance and sustainability.